Wednesday 11 September 2019, at the Scottish Parliament
In conjunction with Reform Scotland, the Futures Forum held a seminar with writer and journalist Tom Chivers on artificial intelligence.
The seminar covered how AI is developing and changing our lives and the way we should approach it as citizens and how the Scottish Parliament can respond as a legislature.
Chaired by Clare Adamson MSP, Convener of the Scottish Parliament’s Cross-Party Group on Science and Technology, the seminar was attended by MSPs, their staff and Parliament staff.
Podcast
Listen to a podcast of Tom’s presentation.
Presentation Transcript
I want to start by talking about what we mean by artificial intelligence, or AI. The word “intelligence” can send us down the philosophical route of considering whether something is truly intelligent and what artificial intelligence really is. However, there are blurred lines—is a machine AI or is it just doing statistics, or is it just software or technology?
A lot of the time, we end up in a philosophical debate, but that can be sidestepped quite easily. The only important question is whether a machine is capable of doing something that previously only humans could do.
People often avoid using the term “artificial intelligence”—they prefer to talk about machine learning, technology or automation, which avoids the whole question of what intelligence really is. To a large degree, however, we don’t care about that question unless we are philosophers—as admittedly I was for a long time. We don’t care what intelligence truly is or if computers know what love is or have emotions—all that sort of stuff. We just want to know whether a machine is competent and powerful, and whether it is doing the things that we want it to do or doing other things.
Over the past few years, AI has become dramatically more competent, and it is now able to do many more human jobs. Simple and repetitive human jobs have been steadily replaced by artificial technologies for a long time, going way back to the Spinning Jenny in the 18th century. However, increasingly, more jobs that we would think of as creative or human jobs are going.
From my point of view, it is mildly unnerving that some forms of journalism can now be automated. Simple financial stories about whether a particular stock has risen or fallen can now be written by algorithms. They do the job with no style or élan, but it is done effectively.
Increasingly, AI can do things that stray into areas that we would consider to be creative. Earlier this year, OpenAI released a model called GPT-2. It was given a line of text by Tolkien as a prompt, and it churned out a thousand words of bad Tolkien fan fiction, although I’ve definitely read worse Tolkien fan fiction by humans.
Only a few days ago, a team—it may have been from Microsoft—managed to build an AI system that could pass an US eighth-grade science test. That involves putting together things such as logical concepts and understanding natural language, all of which push at the idea of what it means to be intelligent.
All the time, we say, “Oh, sure—AI can do this, but it can’t do that, which is the thing that makes us human.” Increasingly, however, AI is eating away at all those areas, because it can do more and more of those things.
There is an important point about the way that AI systems are made. Most modern AI runs on the idea of a neural network, which is to say that it learns through the nodes within it. I won’t go into the details—to put it simply, the nodes get different weightings depending on the data that comes in, so the AI is trained on data.
Essentially, we can think of it in this way: you get vast amounts of data and put it into a black box; it sloshes around a bit and comes out the other end and gives you the outcomes, and you see how it does: whether or not it is good at translating the text you gave it, or at writing Tolkien fan fic.
If the results look really good, that is great, but you don’t know how the AI is getting those results. The risk, therefore, is that the technology will go wrong in some weird way that you won’t know about, because you don’t know what’s going on inside it.
You also won’t know whether your dataset might turn out to be biased in some unpredictable way. That has happened several times. For example, last year, Amazon realised after a while that it had to shut down its hiring algorithm, because all the hiring data that it had been trained on had been skewed towards hiring white men. AI is always a bit of a black box, and it is only ever as good as the data it is trained on.
Jobs and automation
I figured that you guys would be interested, from a governing point of view, in key areas such as the impact of AI on jobs and automation. As I said, the history of that goes back 300 years or so—we are talking about machines replacing humans in jobs going all the way back to the Industrial Revolution. History has shown that there is generally some short-term disruption in which people really do lose their jobs, but new jobs are created—we are not all just failed weavers these days.
Increasingly, AI allows us to augment rather than replace jobs. One example that comes up again and again when I speak to people is the job of a radiologist. Much of a radiologist’s job involves boringly going through scans and saying, “Does this look like it’s cancerous?” AI is now increasingly capable of doing that work, so a radiologist can spend less time boringly going through scans and more time doing what humans are good at, which is spending time with other humans.
It also increases accuracy. If a radiologist who looks at a scan is 90% accurate at judging whether it shows a cancer, and AI gets about 90% right as well, we end up at around 99 per cent accuracy if they both look at it, because the AI and the radiologist make different mistakes. We are saying that the two can work hand-in-hand, rather than saying that we’ve suddenly got AIs coming in and we don’t need radiologists any more.
We can use AI to improve the jobs that people do, and to free up time so that humans can do the things that they, as humans, are more skilled at.
AI also lets us do things that we couldn’t do in the past. I’ve been talking to a lot of people about the use of AI in scientific research. When scientists look at enormous datasets in genome-wide association studies, there are millions and millions of datasets that they have to cross-reference in millions and millions of ways, and they end up with vast datasets that have more combinations than there are atoms in the universe. It would be simply impossible for even the most powerful computer to look through all the possibilities.
With AI, it is rather like chess—it can prune down all the possibilities so that scientists can say, “This stuff isn’t helpful—we don’t need to look at that. We can look at these other areas and possibilities instead.” The technology allows people to look at vast new datasets, which it would previously have been impossible for them to do.
AI can be used in ways that improve the things that we do; it can make us able to do new things; and it can free up humans to do things that they previously couldn’t do.
Generally speaking, there has been a long term tendency for new technologies like the Spinning Jenny to come in, disrupt the economy, put some people out of work—which is really hard on those people—and build new economic structures. There is a concern that AI will be different because, unlike the Spinning Jenny, it is not replacing one single thing. AI has the ability, if it is intelligent, to do absolutely everything that humans can do.
Some people think that the Spinning Jenny is the wrong analogy, and that AI will be more like the automobile. In the early 1900s, the horse was the most important transport technology. It was everywhere, but suddenly the automobile replaced it and New York City went from having tens of millions of horses to a few horses working on novelty farms.
There is a fear—which is probably overstated, but which we cannot avoid—that AI will end up replacing human work. That will present a serious challenge to policymakers, as more and more people find themselves moved out of the job market.
I worry that that fear is overstated and hyped up, but it is possibly a real risk. Even if the real risk is overstated, all technologies throughout history, including the Spinning Jenny, really have been putting real people out of work for some time.
Even if, 10 years or 30 years later, those people have retrained, and they—or their children—have found new work, the change still means many years of economic hardship for real people. That is something that we can seriously expect. In the past, technology moved a lot slower than AI is moving now, so things will change much faster.
Trust in politics
Moving away from jobs and automation, I thought that the politicians here would be interested in the issue of trust in politics. There has been a lot of talk lately about deep-fake videos, which are almost photo-realistic in a way that is very hard for people, or even other AI systems, to detect. They can make people look like they are saying or doing things that they absolutely didn’t say or do.
There is a marvellous one in which Barack Obama calls President Trump an “absolute dips**t” or something like that, if you’ll forgive my language. There was also a video that supposedly showed Mark Zuckerberg saying, “I’ve got everyone’s data and I’m going to do what I want with it.” They can make people say anything they want.
I mentioned GPT-2 earlier, which raises the issue of bots that can create realistic text. Again, it could be really worrying if we think about Twitter bots or people on Facebook who are out there spreading misinformation.
Those issues are worth worrying about, and there is another side to consider: it is not just the fake that is the problem, but the ability to say that something has been faked. If President Trump were to be caught on video again saying things about grabbing women—which happened in 2016, before the real rise of the deep-fake video and before we all became aware of it—it would now be much more plausible for him to say, “It’s a deep fake—I didn’t say that.”
The technology will provide people who are caught in awful situations with an opportunity for plausible deniability, and there is a serious concern that it will reduce overall trust in the system.
There are also key issues relating to privacy and data. Openness and the ability to use data is fantastic and brilliant. For example, it is good if NHS data is made more available to AI firms like DeepMind or is made generally available for researchers to use, as it is an amazing dataset that enables people to do wonderful research.
I recently spoke to some people who are trying to use brain scans to predict the growth of Alzheimer’s disease. They look at the likelihood that someone who has certain features in their brain scan at age 50 will have Alzheimer’s by age 70. The trouble is that AI works on huge amounts of data. Things such as amazing facial recognition software and translation software work by having billions and billions of data points.
In a sense, AI learns very slowly—we could learn much more quickly with that number of data points, but AI learns on billions. When scientists are dealing with things like scans, they are lucky if they have more than a few hundred or few thousand data points. Making those scans more available to researchers would have huge benefits, because it would mean that we would be able to do much more efficient and effective scientific research. However, the more we open up such things, the more likely it is that the data will be leaked.
Theoretically, a lot of the data is anonymised, but it can be de-anonymised fairly easily in a few simple steps. For example, if a 65-year-old guy was referred by a particular GP to a particular hospital for a particular condition, someone could, even if the information didn’t include the patient’s name or date of birth, reverse-engineer the data and work it out by getting the numbers down to a few specific individuals.
There is also a real risk that the data will end up in the hands of people who will use it for purposes that we don’t like—for example, insurance companies could use it to up their premiums and that sort of stuff.
Those are real concerns. There is a genuine—and, as far as I can see, unavoidable—trade-off between the advantages that sharing data brings and the risks that it presents, and that is going to involve a decision that we need to face up to. There will be losses and problems whichever way we go.
What should we do next?
My next bullet point says, “What should we do next?” To be honest, I don’t know, but here are a few thoughts. First, as I said, we should be really careful and thoughtful about where data sharing is good and important and where we should and shouldn’t do it. We need to make it clear to people that they can opt out of sharing data, even if that comes at a cost. People should feel that they have ownership over their data; they should be able to say, “Look—this is my data. I’m aware that it can do good, but I don’t want it out there”, especially in relation to NHS and health data. That should be absolutely fine.
On the economic impact of automation on jobs, my feeling—this point may be political rather than analytical—is that we need to come up with ways of taxing a modern high-tech economy that will allow for redistribution to the people who are left behind by it. I will leave the specifics of that to you guys, but it strikes me that that is going to be important. Some people will be severely left behind by all this. As technology makes the world richer, we should be prepared to look out for the people who fall through the cracks.
Issues such as trust in politics will become really difficult, and there will be good reasons not to trust what we see and read. People aren’t very good at working out what is real and what is not, especially older people—that sounds awful but it is true, as has been shown repeatedly. Younger people are better at that than older people, as they have grown up around technology and have worked out ways of deciding what to trust. An AI expert once told me that it is actually very hard to tell the difference online between a Russian bot and a British pensioner. We have to find ways of warning people that a lot of stuff can’t be trusted, while also saying that that doesn’t mean that they shouldn’t trust anything. There has to be some way to find a way through.
I realise that that is very vague—I am saying, “You should find an answer”, but I don’t know quite what the answer is. Fundamentally, things are changing really fast. Government is by its nature quite conservative and slow-moving—rightly so—and it doesn’t change very fast, as it has to deal with a lot of stakeholders and other things. It may be hard for legislation to keep up in an area that is changing so fast—so good luck with that, I guess.
Speaker biography
Tom Chivers worked for the Daily Telegraph from 2007 to 2014, and was a science writer at BuzzFeed UK from 2015 to 2018. He has received several awards for his journalism, including the ‘Explaining the facts’ category in the Royal Statistical Society’s Statistical Excellence in Journalism awards, and he is the author of the recent book “The AI Does Not Hate You”.
Event partners
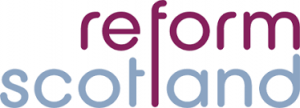
Reform Scotland is a public policy institute which works to promote increased economic prosperity and more effective public services based on the principles of limited government, diversity and personal responsibility.